Wenst u een activiteit te laten opnemen in deze lijst? Geef uw activiteit door via dit formulier.
Artificial intelligence to decode the peptide sequences driving the immune response
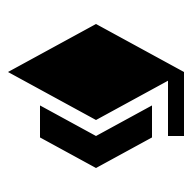
Categorie
Doctoraatsverdediging
Date
2024-09-18 16:00
Locatie
Universiteit Antwerpen, Stadscampus, Gebouw C, lokaal C.002 - Prinsstraat 13, 2000 Antwerpen
Belgie
Belgie
Promovendus/a: Ceder Dens
Promotor(en): Kris Laukens, Wout Bittremieux
Understanding the immune system is of utmost importance for advancements in biomedical research and human healthcare. Deep learning has the potential to uncover the hidden secrets of proteins and peptides, leading to significant improvements in diagnostics, vaccine development, and cancer therapies. In this thesis, we developed and applied various deep learning techniques to get insights from peptides that drive the adaptive immune response.We investigated pathogen recognition and immune response by looking into T-cell receptor and epitope interactions. We apply interpretable deep learning to interaction prediction models and link this to the three-dimensional structure of the molecules to get insights into the factors determining T-cell–epitope binding affinity. Additionally, our results show the importance of using interpretability techniques to verify machine learning models and avoid that small hard-to-detect problems can accumulate to inaccurate results.
We address the issue of data bias in machine learning. We show how it can lead to overly optimistic performance that cannot be reproduced on real-world data. We underscore the necessity of rigorous data evaluation and advocate for the use of unbiased benchmarking datasets to ensure generalizability and applicability of prediction models.
We develop a novel transformer-based machine learning model for interaction prediction of biological sequences. This architecture encodes the interaction between protein or peptide sequences in a biologically meaningful way while also providing valuable visualizations for model interpretability.
We end by focussing on boosting the identification rate of peptides, such as those recognized by the immune system. We find that the training dataset size can have a substantial impact on the performance of machine learning models used in computational proteomics. This underscores the necessity for high-quality, comprehensive, standardized datasets to train robust machine learning models. To address data scarcity, we explore algorithmic strategies such as self-supervised pretraining and multi-task learning. We find that self-supervised learning can be a very valuable technique and hypothesize that the benefits of multi-task learning could become more apparent when used in combination with more comprehensive datasets for all peptide properties.
Artificial intelligence shows great potential to revolutionize biomedical research, however, as shown in this thesis, large, unbiased, high-quality datasets are required for it to make an impact. The findings of this research can hopefully make progress towards more accurate, interpretable, and reliable predictive models, which are crucial for future breakthroughs in diagnostics, therapeutic development, and personalized medicine.
Alle datums
- 2024-09-18 16:00
Powered by iCagenda